Nov 21, 2023
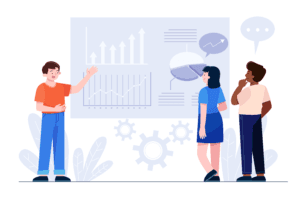
Data lake and data warehouse are two different methods of storing data. While they are similar in their function of storing data, both are used for completely different purposes with varying features.
In this blog post, we’ll go over the key differences between a data lake and a data warehouse and the core features of each.
Let’s get started 🚀
What is a Data Lake?
A data lake is a pool of data, much as the name suggests, it stores data in an unstructured or semi-structured form. No amount of processing or data management is necessary to store data in a data lake. As a result, data lakes require a vast amount of storage to store these varying types of data in their native uncompressed form.
However, data lakes always require some maintenance to make sure that it doesn’t turn into data swamp where data is of poor quality and almost unsuitable to use.
It’s almost impossible for an individual to comprehend data from a data lake without any experience in data science. That’s why only data scientists and analysts deal with a data lake and break it down into clear, concise, and easy-to-understand chunks of data, which leads us to our next topic.
What is a Data Warehouse?
A data warehouse is the home of structured, properly processed, and managed data that can be easily interpreted without any requirement of expertise in data science.
Data warehouses are the perfect solution for businesses and organizations having a goal to interpret data and gain insight from it. The warehouse storage system gives an excellent multi-dimensional view of atomic and summary data.
As a result, tasks like data extraction, cleaning, transformation, and refreshing are easily performable.
The 4 Key Differences Between Data Lake and Data Warehouse
It is clear from the above sections that however similar functions these two serves, they are inherently different in their purpose. So let’s have a look at the differences between a data lake and a data warehouse.
Data Structure: Raw vs Processed
The key difference between the two is the nature of the data they store. Data lakes are used for storing a vast chunk of unprocessed, unfiltered, and unstructured data that may or may not have some specific purpose for later.
Much like an actual lake, where anything and everything can be found at its bottom, a data lake serves the same purpose for storage.
Whereas data warehouses are indeed warehouses as everything about it is structured and processed. No data without a purpose can be found in a data warehouse because the primary goal of a data warehouse isn’t the storage of data but the interpretation and analysis of data.
As a result, most small to mid-scale businesses use data warehouses, whereas big data businesses like Meta or Google rely on data lakes.
Purpose of Data: Undefined vs Defined
In a data lake, data is open to exploration and scrutiny to understand whether the data is useful for immediate purposes or not. Even if some data has no immediate use cases, it is stored for future possibilities and operational use cases.
On the other hand, data warehouses are maintained with the specific goal of data usability. If a dataset serves no purpose in understanding a specific situation, it’s excluded.
Data Timeline: Old vs New Data
Data lakes are maintained to preserve and store thousands of datasets over decades. Even if data isn’t useful or important, data lakes retain the data for possible future use cases. It also retains old redundant data that can possibly serve as comparable perspectives to data professionals.
In data warehouses, most of the data used are updated and latest to have the best insight into the current market condition. Old data are only used when the specific purpose is to understand historical scenarios or learn from past events.
Users: Data Professionals vs Business Professionals
Data lakes are complex stores of data that stores everything in its native versions in thousands. As a result, it’s inherently redundant and even useless in some cases for immediate purposes.
That’s why data professionals (i.e. data scientists and analysts) go through these chunks of data, derive insights, and extract the useful data to data warehouses. Business professionals or government bodies without experience in data science will find data lakes extremely difficult to decipher.
Once the data is analyzed, processed, and portrayed visually with graphs and charts in a data warehouse, it becomes easy to understand for business professionals. As a result, businesses and other organizations always prefer data warehouses.
Bonus: Access Flexibility
Since data lakes aren’t structured or maintained with great precision, it’s extremely easy for anyone to access the data, change, or manipulate it. The vastness of data lakes makes it impossible to check for data integrity. But since warehouses are structured following proper rules and regulations, it’s difficult to access or manipulate without detection.
Data Lake or Data Warehouse: Which is Appropriate for What?
As we have concluded that these two serve two particular purposes, let’s understand what are the real-world use cases of each of them.
The value of big data in recent years has become imminent to our society. It affects all parts of human life including health, education, careers, entertainment, etc.
These big data firms buy data from small hubs such as apps, websites, SaaS platforms, etc. Depending on the type of data, it gets stored in data lakes and then processed into data warehouses and goes off to other businesses who would find those data useful.
Now depending on the nature of your business or organization, you would either go for a data lake or data warehouse. For example, if you’re in education or health, data lakes make more sense, whereas, in finance or business development, data warehouses will serve the best purpose.
Wrapping it Up
Data is the currency of the modern world. Following this analogy, think of data lakes as gold mines and data warehouses as gold storage. After mining thousands of tons of sand and stones, miners get a few milligrams of gold. Similarly, amid millions of data sets, data scientists look for patterns, behaviors, historical records, anomalies, and events to gain some insights.
Data lakes and data warehouses serve two very specific purposes and aren’t interchangeable. Without having proper data lakes to mine through, there won’t be any data warehouses to store data in a structured and orderly manner.
Get Sherloq Free